What is the critical data point crucial for accurate analysis and strategic decision-making? A key element in data-driven methodologies, this specific point provides foundational insight.
A specific data point, often a numerical value or categorical identifier, is a single, discrete observation within a larger dataset. It could be a customer's age, a product's sales figure, or a specific attribute. This single piece of information, when considered in relation to other data points, can reveal patterns and trends. For instance, analyzing the average purchase price of a product alongside the age of the buyer could identify target demographics or inform product pricing strategies.
The importance of this data point lies in its contribution to comprehension. Identifying crucial insights from a multitude of data points allows for strategic decision-making. Analysis of historical trends using such points fosters prediction capabilities, which, in turn, can drive proactive initiatives. These are essential tools for a variety of industries, from finance and marketing to healthcare and scientific research, all depending on data-driven models for efficiency and growth.
Moving forward, we can examine specific applications of this concept within the field of business analysis and data science. Understanding the significance of individual data points allows for a deeper, more accurate grasp of complex relationships within a set of data, leading to powerful outcomes.
Data Point
Understanding the characteristics of a single data point is crucial for accurate analysis and informed decision-making. Reliable data analysis hinges on recognizing and interpreting individual data elements.
- Precision
- Accuracy
- Relevance
- Context
- Representation
- Consistency
- Interpretation
- Validation
These aspects, when considered collectively, determine the validity and utility of a data point. Precision and accuracy are fundamental; a precise measurement of sales figures, for instance, must be accurate to be meaningful. Relevance ensures the data point directly addresses the question or problem being analyzed. Context, encompassing the surrounding circumstances, provides vital background for proper interpretation. Consistent data points, across various datasets, enable reliable patterns to emerge. Careful interpretation converts raw data into insightful information. Validation ensures the data's reliability and trustworthiness. For example, if comparing sales figures across regions, the data must be consistent in methodology to avoid misleading conclusions.
1. Precision
Precision in data collection and analysis is paramount for deriving meaningful insights from a specific data point. A precise data point, free from significant error, forms a solid foundation for subsequent statistical analysis. Without precision, conclusions drawn from data analysis may be inaccurate and misleading. Consider a medical study aiming to determine the effectiveness of a new drug. Precise measurements of patient outcomes, such as blood pressure readings, are critical for assessing the drug's impact accurately. Inaccurate readings or inconsistent methodologies render the study's conclusions unreliable, potentially jeopardizing patient safety and research integrity.
The importance of precision extends beyond scientific research to numerous fields. In financial modeling, precise data on market trends and economic indicators is crucial for accurate predictions and investment strategies. Similarly, in manufacturing, precise measurements of component dimensions ensure product quality and consistency. The consequence of imprecision in these sectors can be substantial, ranging from financial loss to safety hazards. A slightly off-target missile, for instance, demonstrates the dramatic consequences of a lack of precision in critical systems. Accurate and precise data capture is foundational to data-driven decisions in any field.
In summary, precision in data points is fundamental to the validity and reliability of any analysis. Errors in measurement, whether due to flawed methodology or equipment malfunctions, directly impact the accuracy and usefulness of the data gathered. Cultivating a precise approach to data collection ensures that decisions made based on this data are well-founded and minimize potential risks. This underscores the significant need for robust methodologies in data handling and the rigorous application of these principles in every stage of analysis.
2. Accuracy
Accuracy in data points is fundamental to the reliability of any analysis. A data point's accuracy directly impacts the validity of conclusions drawn from it. Inaccurate data points lead to flawed analyses and, consequently, potentially detrimental decisions. Consider a manufacturing process relying on precise measurements of component dimensions. Inaccurate measurements of critical tolerances can lead to product failure and costly recalls. Similarly, a financial institution relying on inaccurate market data might make poor investment decisions, resulting in significant financial losses.
The accuracy of a data point is contingent on various factors. The method used to collect the data plays a crucial role. For instance, using faulty equipment or employing an inadequate sampling technique will introduce error. Data entry errors during the collection or recording process also compromise accuracy. Furthermore, the context in which the data point is situated matters. A data point relevant in one context may be irrelevant or misleading in another. Therefore, careful consideration of the source and context of the data point is essential for maintaining accuracy.
Maintaining accuracy is vital for sound decision-making across diverse fields. In healthcare, accurate patient data drives effective treatment plans and minimizes potential risks. In scientific research, the accuracy of data points underpins the validity of findings. In essence, ensuring data accuracy is not just a technical consideration; it's a crucial component of responsible data handling and ethical analysis practices. The potential consequences of inaccurate data range from simple errors in calculations to catastrophic failures in complex systems. Ultimately, the inherent value of accurate data points hinges on the reliability of the conclusions and subsequent actions derived from their analysis.
3. Relevance
Relevance of a specific data point, often referred to as an "sd point," is critical to effective analysis. A data point's value hinges on its alignment with the specific question or problem being addressed. Irrelevant data points, even if accurate and precise, contribute little to understanding or decision-making. This facet explores how relevance shapes the utility and impact of data points in various contexts.
- Contextual Alignment
A data point's relevance is fundamentally tied to its context. A sales figure for widgets in the United States is highly relevant to an analysis of the US widget market but less relevant to the Indian widget market. Understanding the appropriate context ensures the data point is appropriately considered. Misinterpreting context can lead to erroneous conclusions or wasted effort. For instance, if a marketing team uses data from a competitor's promotion during the summer months to inform their winter promotion strategy, the data's relevance is lost due to context mismatch.
- Problem Definition
The core problem or question guides the selection of relevant data points. If a company wants to understand customer satisfaction, data points like purchase history, customer support interactions, and product reviews are directly relevant. Data on employee turnover or warehouse inventory, while potentially valuable, is likely irrelevant to the specific problem. A clear definition of the issue at hand is crucial for selecting the most relevant data points.
- Data Source Validity
The trustworthiness and reliability of a data source are intricately linked to relevance. If the data originates from a biased or unreliable source, its relevance diminishes, regardless of its apparent alignment with the analysis. Data points from a biased survey, for example, may appear relevant on the surface, but the inherent bias undermines its actual value. A comprehensive assessment of the source and methodology used to gather the data ensures its validity before using it for analysis.
- Data Integrity & Completeness
Data points must be complete and free from inconsistencies to be relevant. Incomplete or contradictory data creates ambiguity and hinders informed decision-making. In market research, for example, a survey with a low response rate or incomplete responses significantly diminishes the data's relevance and trustworthiness, despite being collected from customers. The data's completeness and integrity are prerequisites for its relevance. An incomplete or inaccurate data point, even if aligned to the question, weakens its significance.
Ultimately, the relevance of any data point hinges on its connection to the specific question or problem being examined. A clear understanding of context, problem definition, data source validity, and data integrity directly affects the data point's value and the reliability of subsequent analysis and insights derived from it. Ignoring these aspects undermines the utility of even the most precisely measured data points, ultimately diminishing the overall value of any analysis based on them.
4. Context
The context surrounding a data point, often referred to as a specific data point ("sd point"), profoundly influences its meaning and value. Context provides the necessary framework for interpreting the significance of a single data observation within a larger dataset. Without context, a data point can be misleading or even meaningless. Consider a daily temperature reading. A 70-degree reading in the Sahara Desert holds a vastly different significance than a 70-degree reading in a temperate climate. The geographic locationan element of contextalters the interpretation of the numerical value.
Contextual factors encompass a wide array of elements, including the time period, geographic location, demographic characteristics, and the specific methodology used to collect the data. In financial analysis, for instance, the context of a rising stock price must consider prevailing economic conditions, industry trends, and company-specific factors. A high stock price during a period of economic growth carries a different implication than the same price during a recession. In healthcare, a patient's blood pressure reading needs context such as their age, physical activity levels, and current medications. Without this background information, interpreting the reading becomes problematic and potentially dangerous.
Recognizing the crucial role of context in evaluating data points is paramount. Ignoring or misinterpreting context can lead to flawed analyses and poor decision-making. In business strategy, neglecting the cultural context of a foreign market can result in misinterpreting consumer preferences, leading to failed product launches or ineffective marketing campaigns. In scientific research, neglecting the conditions in which an experiment is conducted can compromise the validity of the results. Therefore, the ability to discern and consider the relevant context surrounding any data point is critical for making accurate inferences and sound decisions. Ultimately, a deep understanding of the "context" of an "sd point" is essential for meaningful interpretation and application of any data in practical settings.
5. Representation
Accurate representation is fundamental to the integrity of any data point. A data point's value stems not only from its inherent numerical or categorical value but also from its faithful representation within the larger dataset. A flawed representation, even if the data itself is accurate, can lead to misinterpretations and ultimately, poor decision-making. Consider a study examining consumer preferences for a new product. If the sampled population does not accurately represent the target demographic, the conclusions drawn about consumer preferences will be unreliable. This misrepresentation could lead to wasted resources or missed market opportunities.
Proper representation encompasses several crucial aspects. Firstly, the methodology employed to collect the data must be transparent and unbiased. If the methodology systematically favors certain groups or excludes others, the resulting data will not represent the entire population accurately. Secondly, the data must be free from significant errors in recording or measurement. Minor inaccuracies can accumulate, leading to a skewed representation of the underlying phenomenon. Thirdly, the context in which the data point is situated must be clearly understood. A data point in one context might be misleading in another, and a comprehensive understanding of the context allows for a more accurate representation of the data. Finally, any limitations in the representation should be acknowledged, as recognizing these constraints is essential for interpreting the findings effectively.
In conclusion, accurate representation is indispensable for trustworthy data analysis. By ensuring the data-collection process is rigorous, error-free, and contextually appropriate, analysts can obtain a reliable representation of the phenomenon under investigation. Failure to prioritize representation undermines the very foundation of data-driven decision-making. The ability to identify and account for limitations in representation is equally crucial for interpreting results cautiously and avoiding erroneous conclusions. Consequently, a conscientious focus on representation enhances the overall trustworthiness and utility of any data-point-based analysis.
6. Consistency
Consistency in data points, crucial for accurate analysis, ensures reliable and meaningful insights. A consistent data point, or "sd point," demonstrates stability and reliability, enabling dependable patterns and trends to emerge. Inconsistency, on the other hand, introduces noise and ambiguity, hindering the extraction of valid conclusions. Maintaining consistency across data collection methodologies and processes is essential to producing trustworthy results. For example, consistent measurements of a product's quality across multiple production runs provide reliable data for assessing overall production efficacy.
Consistency is a cornerstone of rigorous analysis. A standardized methodology for collecting data ensures comparability across different sets. This uniformity allows analysts to draw valid comparisons and identify trends accurately. Consider stock market analysis: Consistent data collection methods, tracking the same factors across different companies, enable the identification of market patterns and predict future behavior. Conversely, inconsistent data sources, where some companies are evaluated using different metrics, yield misleading results, creating inaccurate predictions. In scientific experiments, consistency in experimental design is essential to isolate variables and confirm the causal relationship between variables. Inconsistent designs compromise the validity of findings, as the variability inherent in the experiment clouds the desired effect.
Maintaining consistency in data points requires meticulous attention to detail, standardized procedures, and a comprehensive understanding of the underlying data structure. Failure to maintain consistent data points across different contexts can yield erroneous conclusions, and inconsistent methodologies can introduce biases. In practical terms, organizations must establish clear data quality guidelines and protocols for data collection, validation, and interpretation to mitigate these risks. By investing in consistent practices, organizations enhance the reliability of data-driven decisions, increasing the likelihood of favorable outcomes.
7. Interpretation
Accurate interpretation of individual data points ("sd points") is crucial for deriving meaningful insights. Without a precise understanding of the implications of each observation, data analysis becomes a collection of disconnected facts, lacking contextual significance. Interpretation bridges the gap between raw data and actionable knowledge.
- Contextual Understanding
Interpreting a specific data point requires a profound understanding of its context. The same numerical value, for example, can signify entirely different things in distinct situations. A high sales figure, without knowing the market conditions or competitor activity, carries little meaning. Contextual factors, like seasonality, economic trends, or promotional campaigns, are essential to understanding the true significance of the data point.
- Relationship Analysis
Interpretation transcends individual data points, focusing on the relationships between them. Considering how one "sd point" interacts with others reveals patterns, trends, and correlations. Analyzing the relationship between customer demographics and purchasing behavior, for example, helps businesses tailor their offerings. Interpretation is not just about the value of a single point but about its place within the larger dataset.
- Statistical Significance
Interpretation necessitates recognizing the statistical significance of data points. A seemingly unusual value might arise from chance, not representing a genuine trend. Statistical methods assess the probability that a particular finding is not due to random fluctuation, helping analysts determine which data points are genuinely meaningful. Misinterpreting a non-significant data point can lead to misguided strategies and wasted resources.
- Causation vs. Correlation
A key aspect of interpretation involves distinguishing between correlation and causation. Two variables may exhibit a strong relationship but one not necessarily cause the other. Interpreting a strong correlation between ice cream sales and crime rates, for instance, reveals a relationship but does not suggest that one causes the other. Careful interpretation clarifies whether a data point reflects causation or mere correlation.
In summary, interpreting "sd points" involves a deep understanding of the surrounding context, the relationships between data points, their statistical significance, and the crucial distinction between correlation and causation. This holistic approach transforms raw data into valuable insights, enabling informed decisions and strategic actions. This comprehensive understanding of the interpretation process is essential for the validity and utility of any analysis based on specific data points.
8. Validation
Validation of specific data points ("sd points") is essential for ensuring the reliability and trustworthiness of any analysis. It involves confirming the accuracy, consistency, and appropriateness of individual data elements within a larger dataset. Without validation, the insights derived from the data may be flawed, leading to poor decisions or misleading conclusions.
- Data Source Verification
Validating the source of an "sd point" is crucial. Ensuring the data originates from a reliable and unbiased source mitigates potential errors. For example, verifying that a sales figure is from a company's official financial report, rather than an unofficial blog post, provides confidence in its accuracy. This validation step is essential for building trust in the data's reliability.
- Methodological Scrutiny
The methodology used to collect the data must be scrutinized. Validating the methodology ensures that the data collection process was standardized, consistent, and free from bias. For example, analyzing survey data requires validation to ensure the survey questions were clearly worded, the sample size was representative, and the data collection process was impartial.
- Consistency and Completeness Check
Validating for consistency and completeness within the dataset helps identify errors or inconsistencies within the "sd points". A consistent dataset ensures comparable data across different time periods or contexts. For instance, in financial forecasting, ensuring consistent accounting methods across years is essential to accurate projections. A sales dataset should be complete, meaning all expected data points are present.
- Data Accuracy Assessment
Evaluating the accuracy of individual data points is crucial. This process might involve comparing the "sd point" against external benchmarks or other reliable data sources. For instance, validating a temperature reading involves comparing it to readings from a verified instrument or a known standard.
These validation steps are interdependent and form a crucial framework for evaluating the trustworthiness of any data point. Ignoring any of these facets can introduce significant errors or biases into the analysis. Consequently, comprehensive validation procedures are essential for ensuring the integrity and accuracy of any data-driven decision. Rigorous validation safeguards against false interpretations, leading to more robust and actionable insights.
Frequently Asked Questions about Specific Data Points ("sd Points")
This section addresses common questions and concerns surrounding specific data points, often abbreviated as "sd points." Understanding these aspects is critical for accurate analysis and informed decision-making.
Question 1: What is a specific data point ("sd point")?
A specific data point is a single, discrete observation within a larger dataset. It can be a numerical value, a categorical identifier, or any other measurable characteristic. Examples include a customer's age, a product's sales figure, or a specific attribute of a manufactured component. These individual data points, when considered in relation to other data points, reveal patterns and trends, enabling strategic insights.
Question 2: Why is data point validation crucial?
Data point validation is essential to ensure the reliability of any analysis. Inaccurate or inconsistent data points can lead to flawed interpretations and ultimately, poor decisions. Validation checks data accuracy, consistency, and relevance to the analysis's goal. A robust validation process ensures confidence in the data and the derived insights.
Question 3: How does the context of a specific data point influence its interpretation?
Context is paramount when interpreting an "sd point." A data point's meaning is heavily dependent on its surrounding circumstances. For example, a high sales figure might be significant in one market context but not in another. Careful consideration of the time period, location, demographics, and other contextual factors is essential for proper interpretation.
Question 4: What is the difference between correlation and causation when analyzing data points?
While correlation signifies a relationship between two variables, causation implies a direct cause-and-effect relationship. Analyzing data points requires distinguishing between these two concepts. A strong correlation doesn't automatically mean one variable causes the other. Careful interpretation is needed to avoid drawing inaccurate conclusions.
Question 5: How can I ensure the consistency of my data points?
Consistent data points are critical for reliable analysis. Standardized data collection methods, clear definitions of variables, and meticulous data entry procedures are essential for maintaining consistency. This standardization ensures comparable data across different contexts or time periods. Consistent data improves the reliability and validity of analytical results.
Understanding these frequently asked questions about specific data points will provide a more robust framework for analyzing and interpreting data, ultimately facilitating better decision-making in various fields.
Moving forward, we will explore practical applications of these concepts in specific industries.
Conclusion
This exploration of specific data points ("sd points") underscores the crucial role of individual data observations in driving accurate analysis and informed decision-making. The article highlighted the importance of precision, accuracy, relevance, context, representation, consistency, interpretation, and validation in the handling of individual data points. These attributes are interconnected and essential for ensuring the reliability and utility of data-driven conclusions. The analysis emphasized that a single data point's value is contingent upon its context and relationship to other data points within the broader dataset. Methodological rigor and a thorough understanding of the underlying data structure are crucial for obtaining trustworthy results.
The significance of "sd points" extends across diverse sectors, from scientific research and financial modeling to market analysis and healthcare. Accurate and insightful utilization of these individual data points is paramount for the development of robust and reliable analytical models. A commitment to meticulous data handling practices, a deep understanding of the contextual factors influencing individual data points, and a thorough validation process are essential for harnessing the power of these elements. Further research should investigate the application of these principles in emerging technologies, such as artificial intelligence and machine learning, as the need for reliable data analysis is certain to remain crucial in a data-driven future.
South HDHub4U: Latest Movies & Shows!
HD Hub 4 U: Ultimate Streaming!
Best HDHub4U South Indian Hindi Dubbed Movies & Shows!


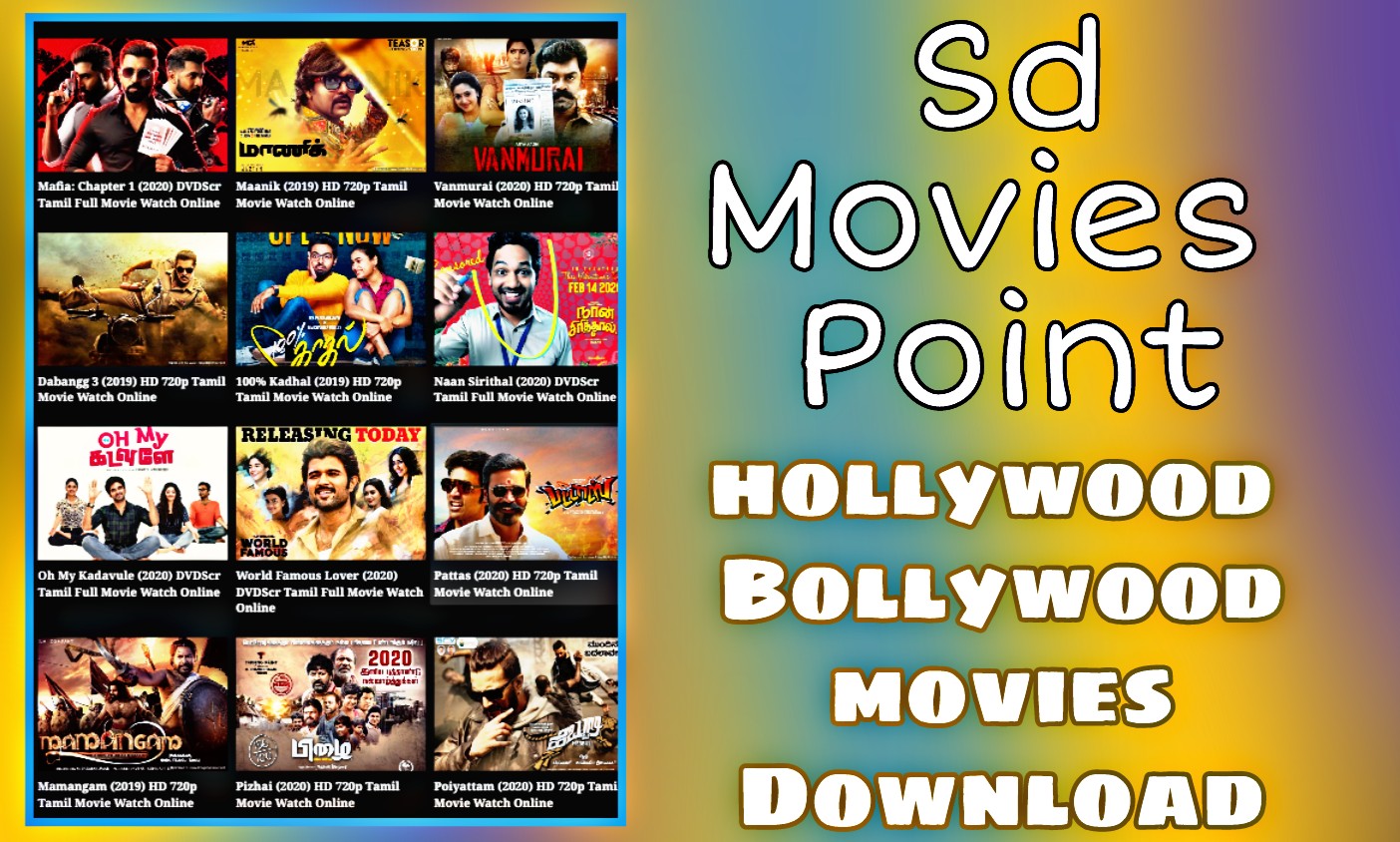